Central limit theorem
Table of Contents
Central limit theorem
A key idea in statistics, the central limit theorem, or CLT, is important in many industries, including banking. It offers a crucial perspective on the behaviour of sample means, making it an essential tool for making decisions and testing hypotheses. Understanding the central limit theorem is essential for analysts, researchers, and practitioners who work with data analysis since it forms the basis for many statistical techniques. Here, we look at CLT, its formula, essential elements, and useful examples.
What is CLT?
The CLT states that, regardless of the shape of the available population distribution, the sampling distribution of the mean of a sufficiently large number of independent random samples from that population will be approximately normally distributed. This remarkable property holds regardless of whether the original population follows a normal distribution.
Understanding CLT
Although the CLT may initially seem difficult to understand, its implications are crucial for understanding how samples behave and are distributed.
Consider a population with a mean and a standard deviation (σ) to understand the CLT. Consider that we randomly select (n) samples from this population, each with a sample size (n). According to the CLT, as the sample size (n) rises, the distribution of sample means will likely resemble a normal distribution with a standard deviation (σ/√n) that is centred on the population mean (μ).
The CLT is a powerful statistical tool, allowing analysts and researchers to conclude population parameters using sample means, even in the presence of non-normal populations.
By understanding the significance of sample size, independence, and its ability to accommodate diverse population distributions, one can harness the full potential of this theorem in making accurate and meaningful inferences from data. As a cornerstone of statistical theory, the CLT continues to underpin various research studies and practical applications across numerous domains, ensuring robust and reliable data-driven decision-making.
Significance of sample size (n):
According to the theory, the distribution of sample means tends to follow a normal distribution as the sample size rises. As a result, more accurate estimations of the population mean may be obtained from samples of greater sizes.
Consider sampling a population as being analogous to repeatedly flipping a coin. The distribution of heads and tails tends to equalise when the number of coins reversed (50% heads, 50% seats). Similar to this, regardless of the population’s underlying distribution, the sample mean approaches the genuine population mean when the sample size is sufficiently big.
Formula
The formula of the CLT is given below:
If X1, X2, …, Xn are independent and identically distributed random variables with a mean μ and a finite variance σ^2, then the sample mean (X̄) of these random variables has an approximately normal distribution with mean μ and standard deviation σ/√n, as n (the sample size) becomes large.
Mathematically, it can be written as:
X̄ ~ N(μ, σ^2/n)
Where:
X̄ = Sample mean
μ = Population mean
σ = Population standard deviation
n = Sample size
Key components of CLT:
The CLT’s key components include
- Sample size (n):
As the sample size increases, the sampling distribution approaches normality. Larger sample sizes yield a better approximation of the normal distribution.
- Independence
The samples drawn must be independent, ensuring one sample does not influence another.
- Population distribution
The CLT does not require the population to be normally distributed. It holds for various population distributions.
Examples:
Let’s have a look at an illustration of CLT. Consider a population with an average IQ of 100 and a standard deviation of 15. Let’s compute the means of several random samples from this population of size 30. With a mean of 100 (the same as the overall mean) and an average deviation of 15/√30, or around 2.74, these sample means will have a roughly normal distribution.
Frequently Asked Questions
CLT is crucial in inferential statistics, where we often work with sample data rather than the entire population. It allows us to infer the population mean using the sample imply and apply parametric tests, like t-tests and Z-tests, to conclude the people.
CLT can be summarised in three essential rules:
- The sampling distribution of the sample means will be approximately normally distributed.
- The mean of the sample means will be equal to the population mean (μ).
- The population standard deviation (σ) divided by the square root of the sample size (n) will give the standard deviation of the sample means.
The central limit theorem is of paramount importance in statistics and data analysis. It provides a foundation for many statistical techniques, allowing us to use normal distribution properties even when dealing with non-normally distributed populations. This simplifies calculations and aids in making more accurate statistical inferences.
The CLT is the cornerstone for several statistical methods, including confidence intervals and hypothesis testing. Predictive modelling, machine learning, and quality control are just some areas where it has applications. The CLT offers a strong and broadly applicable framework for dealing with real-world data and making reliable statistical choices by permitting normality assumptions for sample means.
CLT is a statistical concept that states the distribution of sample means becomes approximately normal, regardless of the population distribution, as the sample size increases. Its conditions include having a sufficiently large sample size, independent samples, and the absence of any skewness or heavy tails in the population distribution.
CLT is a statistical principle stating that when we take many random samples from any population, the average values from these samples will be normally distributed, even if the original population itself does not follow a normal distribution.
Related Terms
- Mark-to-market
- Federal Open Market Committee
- FIRE
- Applicable federal rate
- Assets under management
- Automated teller machine
- Balanced scorecard
- Analysis of variance
- Annual percentage rate
- Double Taxation Agreement
- Floating Rate Notes
- Average True Range (ATR)
- Constant maturity treasury
- Employee stock option
- Hysteresis
- Mark-to-market
- Federal Open Market Committee
- FIRE
- Applicable federal rate
- Assets under management
- Automated teller machine
- Balanced scorecard
- Analysis of variance
- Annual percentage rate
- Double Taxation Agreement
- Floating Rate Notes
- Average True Range (ATR)
- Constant maturity treasury
- Employee stock option
- Hysteresis
- RevPAR
- REITS
- General and administrative expenses
- OPEX
- ARPU
- WACC
- DCF
- NPL
- Capital expenditure (Capex)
- Balance of trade (BOT)
- Retail price index (RPI)
- Unit investment trust (UIT)
- SPAC
- GAAP
- GDPR
- GATT
- Irrevocable Trust
- Line of credit
- Coefficient of Variation (CV)
- Creative Destruction (CD)
- Letter of credits (LC)
- Statement of additional information
- Year to date
- Certificate of deposit
- Price-to-earnings (P/E) ratio
- Individual retirement account (IRA)
- Quantitative easing
- Yield to maturity
- Rights of accumulation (ROA)
- Letter of Intent
- Return on Invested Capital (ROIC)
- Return on Equity (ROE)
- Return on Assets (ROA)
Most Popular Terms
Other Terms
- Adjusted distributed income
- International securities exchanges
- Settlement currency
- Federal funds rate
- Active Tranche
- Convertible Securities
- Synthetic ETF
- Physical ETF
- Initial Public Offering
- Buyback
- Secondary Sharing
- Bookrunner
- Notional amount
- Negative convexity
- Jumbo pools
- Inverse floater
- Forward Swap
- Underwriting risk
- Reinvestment risk
- Final Maturity Date
- Payment Date
- Secondary Market
- Margin Requirement
- Pledged Asset
- Yield Pickup
- Subordinated Debt
- Trailing Stops
- Treasury Stock Method
- Stochastic Oscillator
- Bullet Bonds
- Basket Trade
- Contrarian Strategy
- Exchange Control
- Notional Value
- Relevant Cost
- Dow Theory
- Speculation
- Stub
- Trading Volume
- Going Long
- Pink sheet stocks
- Rand cost averaging
- Sustainable investment
- Stop-limit sell order
- Economic Bubble
- Ask Price
- Constant prepayment rate
- Covenants
- Stock symbol
- Companion tranche
Know More about
Tools/Educational Resources
Markets Offered by POEMS
Read the Latest Market Journal
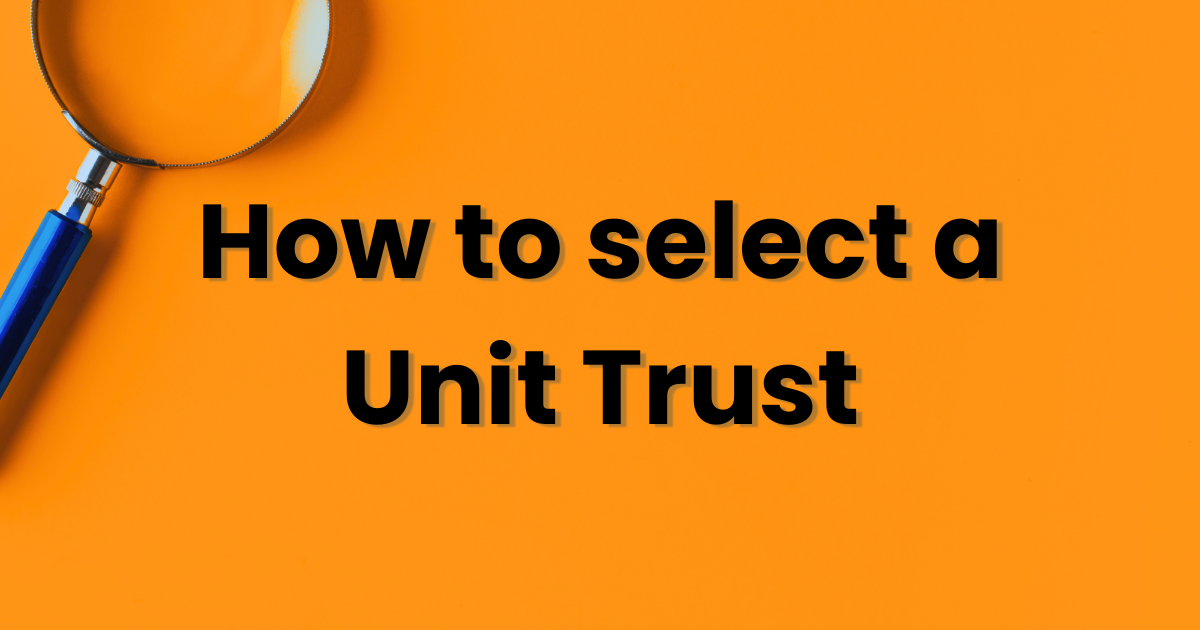
Navigating the vast world of unit trusts can be daunting. With nearly 2000 funds available...

Predicting Trend Reversals with Candlestick Patterns for Beginners
Candlestick patterns are used to predict the future direction of price movements as they contain...
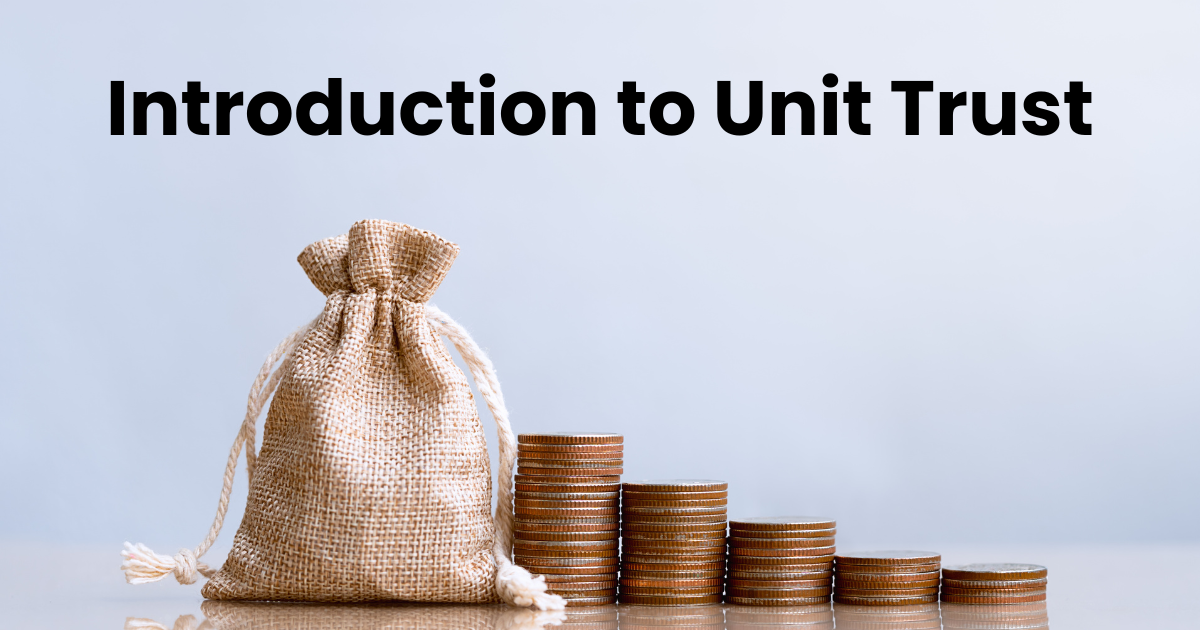
In the diverse and complex world of investing, unit trusts stand out as a popular...
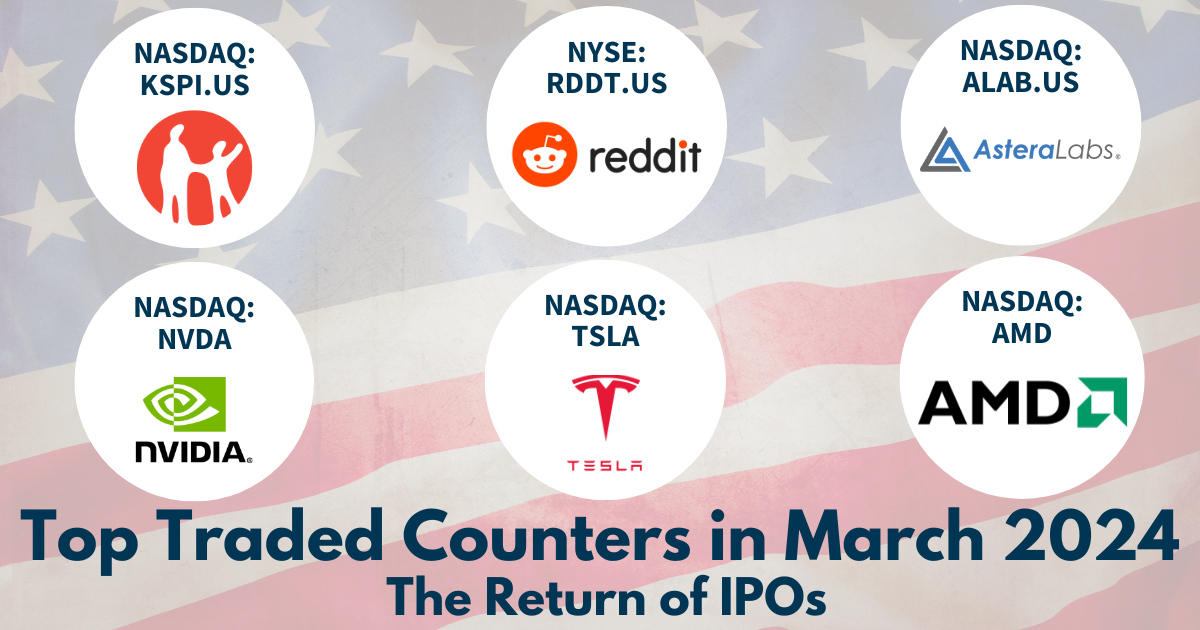
Back in Business: The Return of IPOs & Top Traded Counters in March 2024
Start trading on POEMS! Open a free account here! At a glance: Major indices continue...
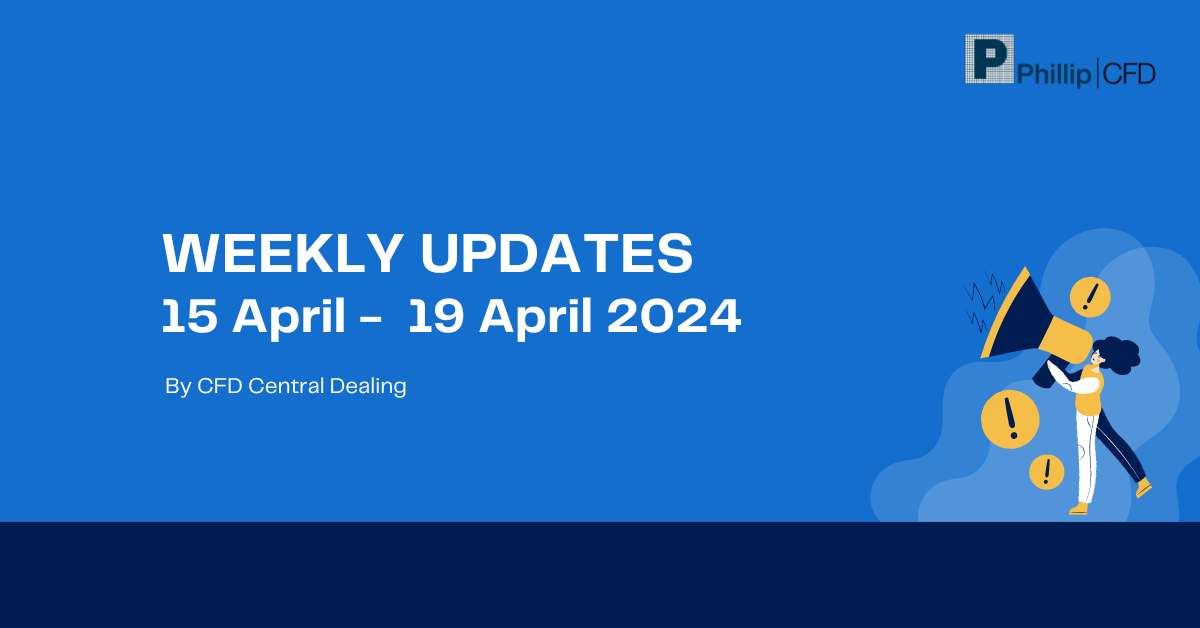
Weekly Updates 15/4/24 – 19/4/24
This weekly update is designed to help you stay informed and relate economic and company...
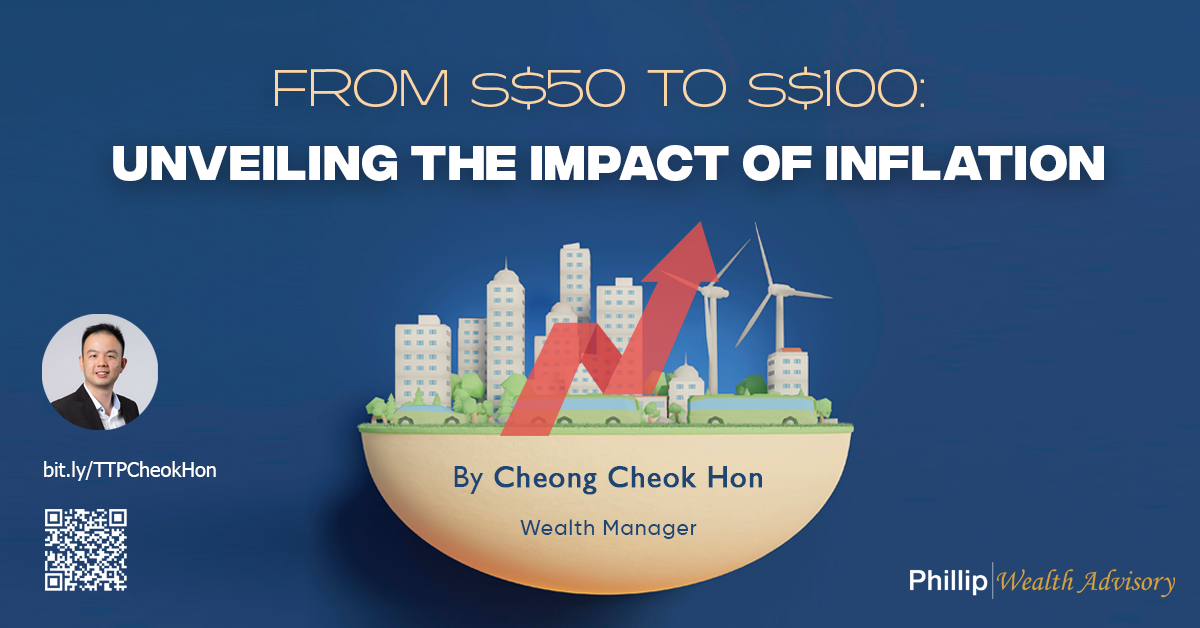
From $50 to $100: Unveiling the Impact of Inflation
In recent years, inflation has become a hot topic, evoking strong emotions as the cost...
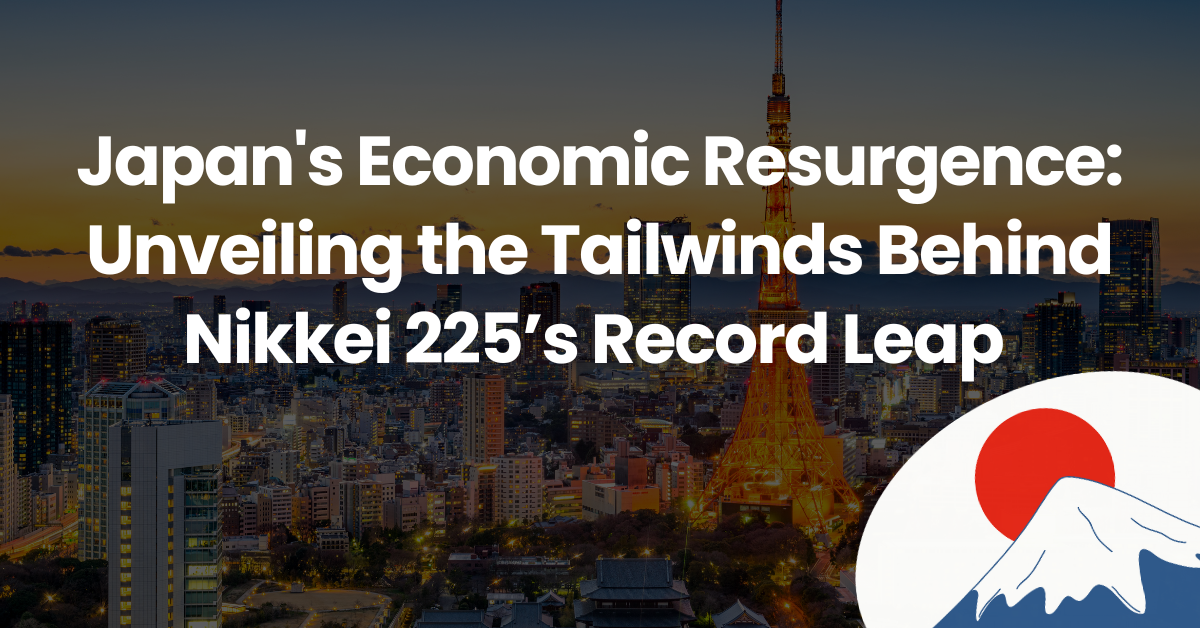
Japan’s Economic Resurgence: Unveiling the Tailwinds Behind Nikkei 225’s Record Leap
Source: eSignal, Intercontinental Exchange, Inc. In the heart of Japan’s economic landscape, the Nikkei 225...
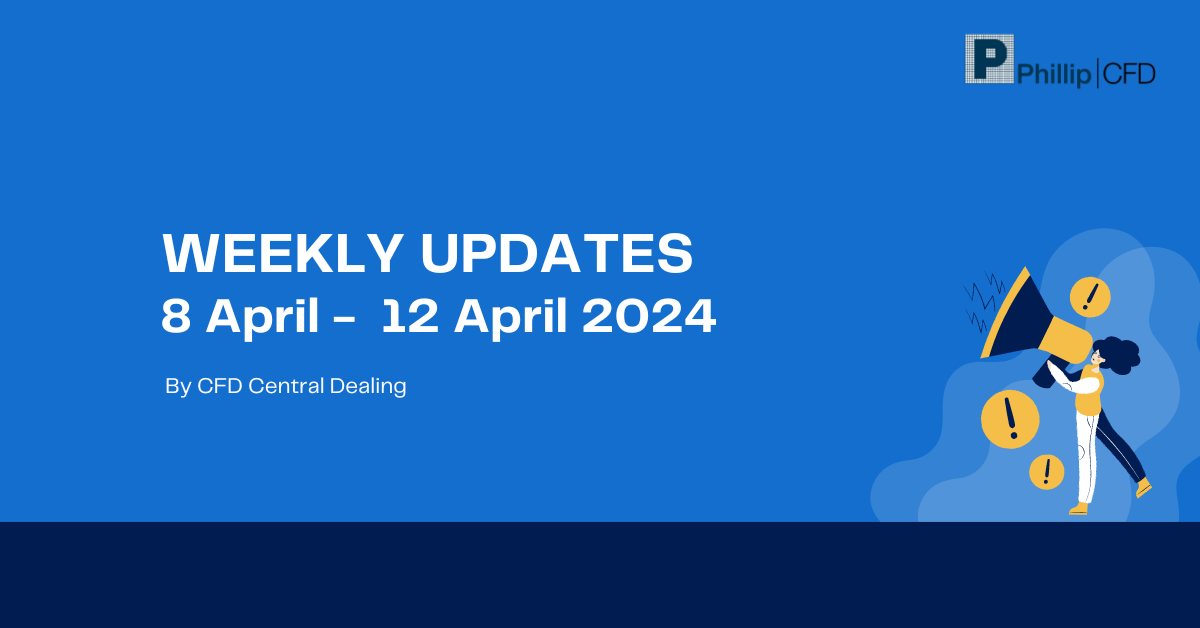
Weekly Updates 8/4/24 – 12/4/24
This weekly update is designed to help you stay informed and relate economic and...